PredictibilityAI predictive analytics:
is the process of using artificial intelligence (AI) and machine learning techniques to analyze historical and real-time data in order to make predictions and forecast future outcomes or trends.
It involves extracting valuable insights from large datasets to identify patterns, correlations, and trends that can be used to predict future events or behavior.
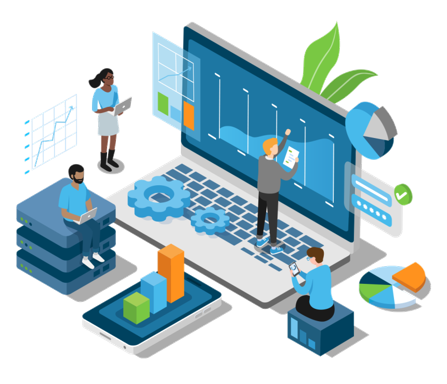
Important features or variables are selected or extracted from the dataset. This process helps to reduce dimensionality and focus on the most relevant information for prediction.
The collected data is cleaned and transformed into a suitable format for analysis. This step involves handling missing values, removing outliers, and normalizing data.
AI models, such as regression, classification, or time series algorithms, are trained on historical data. These models learn patterns and relationships in the data to create predictive models.
The trained models are evaluated using performance metrics to assess their accuracy and reliability. This step helps in identifying the most effective model for making predictions.
Once the models are deemed satisfactory, they are used to predict future outcomes based on new or real-time data. These predictions can be in the form of numerical values, classifications, or probability estimates.
The predictive models are deployed into production systems and continuously monitored to ensure their performance and accuracy. Feedback from the predictions is used to refine and improve the models over time.
AI predictive analysis has a wide range of applications across industries. It can be used for sales forecasting, demand prediction, risk assessment, fraud detection, recommendation systems, quality control, supply chain optimization, and many other areas where accurate predictions can drive business value and inform decision-making.