AI Segmentation in Healthcare:
PredictibilityAI segmentation involves partitioning or segmenting medical images to identify and delineate specific regions or structures of interest. This technique enables the precise localization and measurement of anatomical structures or abnormalities within medical images.
PredictibilityAI:
For instance, PredictibilityAI segmentation can be used to segment and measure tumors, organs, blood vessels, or lesions in medical imaging such as CT scans, MRI scans, or ultrasound images. By providing accurate and automated segmentations, AI can assist radiologists and clinicians in treatment planning, surgical guidance, and monitoring disease progression.
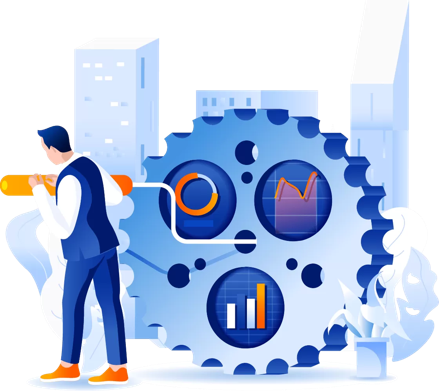
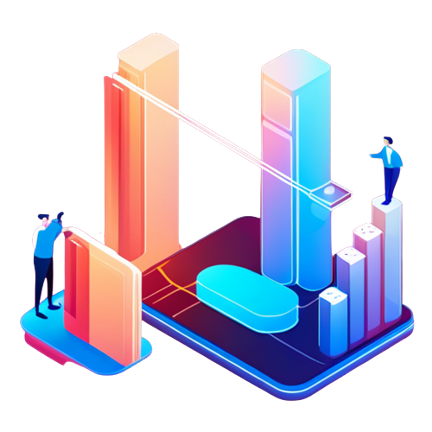
PredictibilityAI:
Segmentation can also be applied to other types of healthcare data, such as electrocardiograms (ECGs) or electroencephalograms (EEGs), to segment and analyze specific signals or waveforms for diagnostic purposes.
PredictibilityAI:
classification and segmentation in healthcare have the potential to improve efficiency, accuracy, and consistency in medical imaging analysis. By leveraging large datasets and powerful AI algorithms, these techniques can enhance the speed and precision of diagnoses, assist in treatment decisions, and support medical research efforts. However, it’s important to note that these AI models should always be used as decision support tools, and final decisions should be made by healthcare professionals based on their expertise and clinical judgment.